Medically Reviewed By: Bradley Bernstein, MD, PhD
The human genome is a sequence of Ts, Gs, Cs, and As that is about 3 billion letters long.
And, says Bradley Bernstein, MD, PhD, chair of Dana-Farber’s Department of Cancer Biology, “there’s no dictionary or guide for reading it.”
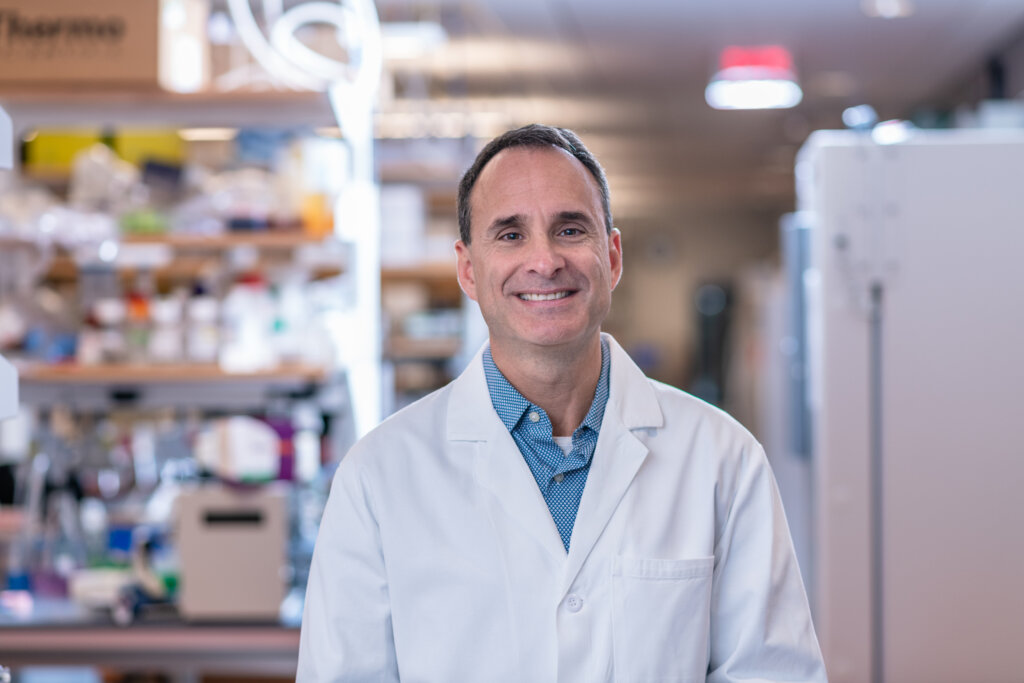
Bradley Bernstein, MD, PhD, chair of Dana-Farber’s Department of Cancer Biology
Bernstein is trying to make such a resource. The efforts so far, carried out by Bernstein and many others, have made important progress. For example, discoveries about the human genome have contributed to advancements in many treatments for cancer, which is often described of as a disease of the genome.
To accelerate this work, Bernstein and first author Nauman Javed, MD, PhD, who was a doctoral student in Bernstein’s lab and is now a resident at Brigham and Women’s Hospital, recently partnered with artificial intelligence (AI) experts at Google to decode parts of the human genome that remain mysterious. The team created a model that is similar to other large-language models, such as ChatGPT, and trained it to “read” the genome and related data in many different cells. The resulting model, called epiBERT, can predict how different cell types use the genome for their specialized functions. The details of the model were recently published in Cell Genomics.
“We really need to understand the genomic code and how it works if we want to advance treatments of diseases like cancer,” says Bernstein.
Choosing AI
Early efforts to decode the human genome identified the sections of the human genome that code for proteins, the machines that do the work inside of cells. Most of the cancer treatments that have emerged from this work specifically target mutant proteins.
The protein-coding sections are the easy ones, says Bernstein. Protein coding regions have recognizable features, so they are easy to identify. Also, when protein-coding genes are missing or mutated, cells malfunction, and the effects can be studied in the lab.
The challenge is that protein coding genes make up only about 2% of the genome.
A large fraction of the remainder is made up of sequences that code for what are called “regulatory elements.” Regulatory elements are responsible for turning genes on or off and setting the volume levels in cells. These regulatory elements are currently poorly understood and unmapped, but they make a huge difference in the workings of the human body.
“Every cell in the body has the same genome sequence,” says Bernstein. “The difference between a brain cell or a liver cell or a skin cell is not which genes you have, it is which genes are turned on, when, and how much.”
Regulatory elements are also believed to hold untapped potential for understanding and treating disease.
“Diseases like diabetes and cancer often involve mutations that are single letter changes in the genome, but few are in protein-coding genes,” says Bernstein. “They are in these other regions, but we can’t interpret their effects if we don’t know what those regions code for.”
For a long time, Bernstein’s lab and others have focused on developing new ways to mutate the genome deliberately, precisely, and efficiently to help understand the regulatory code. In late 2024, for instance, Bernstein’s lab published a paper in Science describing an extremely efficient tool called the HACE tool to contribute to that important work.
“This was an exciting advancement, but we also started to realize that maybe AI could bring this to another level,” says Bernstein.
Training the model
Bernstein and Javed hypothesized that three types of information are needed to train an AI model to make predictions about regulatory elements.
The first is the genome sequence itself. The second is which parts of the genome are accessible.
Genomes are packed into chromosomes inside a cell. Genes and regulatory portions of the genomic DNA can be unwound so they can be read. Previous research from Bernstein and others has resulted in the creation of maps of which parts of the genome are accessible, called chromatin accessibility maps, for many different cell types in many different states.
The third type of data needed is observed measures of which genes are expressed in each cell type and cell state.
Another key decision is what kind of AI model to use. Javed and colleagues at Google selected a model called BERT. BERT is a deep learning model designed to understand and model text. EpiBERT is where epigenetics meets BERT.
“Neural networks are good at dealing with unstructured data and learning underlying patterns by themselves, so it makes sense to apply this kind of model to the genome, where you have a huge genome sequence and a large number of interacting genes and you don’t know the rules in advance,” says Javed.
The team trained the model to learn which of the genome’s regulatory elements influence gene expression across many cell types, building a “grammar” that is generalizable and predictable. This grammar-building process can be likened to the way a large language model, such as ChatGPT, learns to build meaningful sentences and paragraphs from many examples of text.
The EpiBERT model can evaluate a never-before-seen cell and, using the cell’s genome sequence and what is known about its chromatin accessibility, accurately tell the story behind the cell’s function by predicting RNA expression. EpiBERT is also interpretable, meaning it is possible to trace its predictions back, getting a sense of the reasoning and whether the model is accurate.
“It’s a pretty compelling demonstration case for AI in biology,” says Bernstein.
Looking forward
Bernstein is excited about the potential for models like EpiBERT to provide clues that could lead to treatments for cancer. His lab plans to use EpiBERT to learn more about the antigens a tumor cell is expressing, which could point to potential antibody-based therapies for cancer.
The EpiBERT code is shared on GitHub, a hub for software code sharing. But Bernstein notes that training EpiBERT and models like it is still expensive. Bernstein sees this and other limitations around the use of AI, however, as important challenges to overcome because of the potential for AI to accelerate discovery in cancer research.
“We need to empower scientists here at Dana-Farber and elsewhere to benefit from the capabilities of AI,” says Bernstein.
About the Medical Reviewer
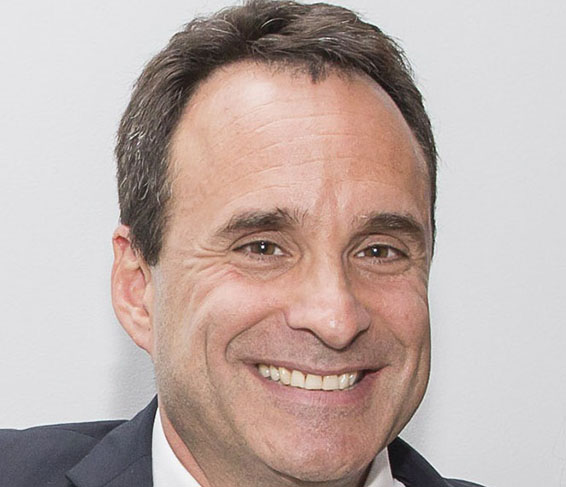
Dr. Bernstein received his B.S. from Yale University in 1992 and his M.D. and Ph.D. from the University of Washington in 1999, before completing a residency in clinical pathology at Brigham and Women’s Hospital and postdoctoral research at Harvard University. He served on the faculty at Massachusetts General Hospital from 2005 to 2021. He is currently Chair of Cancer Biology at the Dana-Farber Cancer Institute, where he holds the Richard and Nancy Lubin Family Chair. He is also the Director of the Gene Regulation Observatory at the Broad Institute, a Professor of Cell Biology and Pathology at Harvard Medical School, and an Investigator in Harvard’s Ludwig Institute.
Written by: Beth Dougherty